Don’t trust your gut! Why data is king
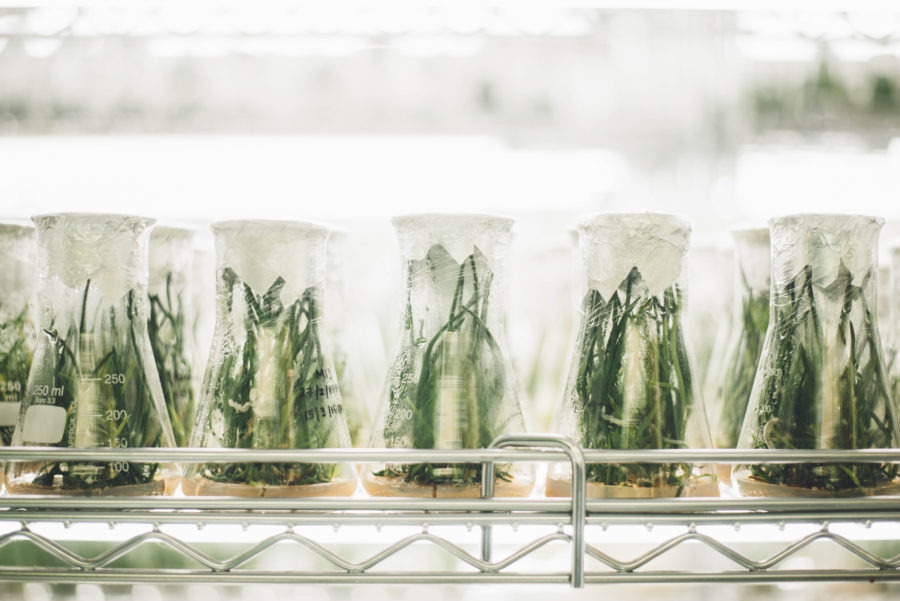
Imagine the following situation: A large toy retailer notices that every time they spend a lot of money on advertising, they sell more toys. They plot their data in a pretty figure and sure enough there is a line showing that when advertisement spending increases sales also increase. They draw the “obvious” conclusion that advertising increases sales. Simple, right? Not so fast! What time of year do toy retailers spend most money on advertising? If they would have looked at the timing of the advertising and the sales, they would have realized that they spend most money on advertising in December! See where this is going? December is also the period where demand for toys is at its peak. Children want toys for Christmas regardless of advertisements and parents will go out and buy them. Now, you might argue, that without advertising the customers will buy fewer or less expensive products. But how would that affect revenue? Is it worth it spending millions on advertising, if the effect on revenue is small compared to the advertising budget?
How can we figure this out? We would need two worlds at Christmas time. One world in which the company runs an advertisement as usual and one in which they don’t, keeping everything else constant. In both worlds we would then observe the revenue from sales and could easily calculate the ROI from advertising.
Luckily this is easy to do. Usually this fictional retailer would send out advertisements to households all over the country. Instead, they now randomly select a fraction of the postal codes in the country who will not receive the advertisements in the mail this year. The rest receives the catalogue as usual. At the checkout in stores, customers are then asked to provide their postal code when making a purchase. At the end of the season, the retailer can now compare whether there were fewer customers from regions that did not receive any advertisements and whether they spent less in the stores. This process is a basic randomized controlled trial (RCT).
With a randomized controlled trial we can create two worlds that are the same, except for one controlled difference – the difference that we are interested in. Why couldn’t we have just sent advertisements one year and no advertisements the next year and compared the difference? Good question! There are so many factors that influence sales that change between years, that we would not have been able to tell whether it was the change in advertisements or perhaps a new game console, a down-turn in the economy, a competitor’s strategy or hundreds of other unobservable differences that affected sales along with our changed advertising strategy.
For a seasoned advertiser, running a randomized controlled trial to see what works best might be old news. However, in our work we still see many organizations measuring effectiveness by comparing last year’s numbers with this year’s numbers or department 1, which is using the old approach to department 2 which is using a new approach. While in some cases this might provide an indication of whether the general strategy of a company is profitable, it doesn’t allow for a causal interpretation of an action.
Ultimately, we want to know: Does X cause Y? Does increasing the advertising budget lead to increased sales? Does the new bonus system increase employee motivation? Does a social norms nudge lead to more recycling?
Correlation and causation
Without a randomized controlled experiment though, we observe only correlation, not causation.
So what is the difference? It really isn’t that difficult, but so many times correlation is mistaken for causation.
Correlation is when I observe two outcomes (such as spending on advertisements and sales) moving at the same time. Spending on advertisements and sales might both go up at the same time or one might go up and the other might go down. We might see department 1 with the old bonus system stagnate in their productivity while department 2 with the new bonus system increases their productivity. From these observations we cannot claim causality. We do not know whether advertisements cause sales to go up and we do not know whether the new bonus system caused department 2 to be more productive. In the retailer case we assumed that it might be the Christmas season that is causing both measure to go up. In the case of the bonus system it could be that in addition to introducing the new bonus system, the department also introduced other measures that caused the increase in productivity.
Correlation: X and Y happen together (and are both caused by Z)
Causation: X has an effect on Y
We need some form of randomization to establish causation. In cases where randomization is not possible, there are fancy sounding, time-consuming methods (instrumental variable approach, propensity score matching, difference-in-difference approach, etc.) to get as close as possible to identifying causation, but for firms, who have the chance to experiment, an experiment is usually the easier way.
Start-ups and programmers have already embraced this method for lean development processes and understanding what works.
RCTs and Behavioral Economics
Conducting randomized controlled trials is especially important when working with behavioral economics and nudging. Not only are the people we are trying to affect biased, but also we, the ones trying to affect other’s behavior, suffer from biases. The human brain is great at seeing patterns were none exist and biases such as confirmation bias, leads us to interpret information in line with what we want to see. So the marketing executive who spent millions on a marketing campaign wants to see in the data that it had the desired effect. Experiments take out the ideology, the opinions, the gut feelings and replace them with empirical evidence. Once you have the cold hard evidence of what works and what doesn’t you can then use your softer skills to decide what to do with it.
In an interview with First Round Gina Gotthilf, VP of Growth at language education platform Duolingo described this very well: “We A/B test nearly everything and focus on whatever produces results. Whatever doesn’t, we don’t. It’s a very straightforward way of looking at things. Instead of leaving decisions to opinion or egos or background, we let the metrics make the vast majority of decisions.”
At Impactually, we use randomized controlled trials whenever possible to understand what works. We know that there is no expert in the world who can say for sure what effect an intervention will have on behavior, but we have a powerful tool to find out the answer.
The days of Don Draper Type gurus, who “just know” which measure to implement, are coming to an end. The question whether some people “just know” has been recently studied by two prominent economists, Stefano DellaVigna and Devin Pope. In their experiment they asked various groups of people to make predictions about whether different measures such as increasing wages or adding a nudge would increase employee motivation. Unsurprisingly, they found that behavioral economists with a PhD were better at predicting reactions to the measures than students, MBAs or people without any formal training in the subject, but there was not a single person who was always right. And this study included award-potentially even Nobel prize-winning behavioral economists (it was anonymized, but it is very likely)! So if even the best in the world with decades of experience are not always right, then let’s all be humble and embrace the process of smart testing over “gut feelings” and “eyeballing”.
Want to hear us talk more about behavioral economics and science? Check out the preview of our online course “Get behavioral science to work for you”, at the bottom of the course page.
You can also download our free and easy-to-use Introductory Guide, which introduces the foundations of the field for people who want to work with behavioral science and nudging in practice.
—
Christina Gravert
Christina.Gravert@impactually.se
+46 76 070 33 89
I want to learn more!
We hear you. If you’ve already read our post about what behavioral economics is, and how it can be used in practice, then here’s a list of books, TED Talks and other online resources. Other interviews, articles and videos we have published elsewhere can be found here. You are also welcome to sign up to our newsletter, where we give you relevant news and links on behavioral economics.
I’m convinced that behavioral economics can do wonders for me. Now what?
Contact us and let’s talk about how we can help you get going.
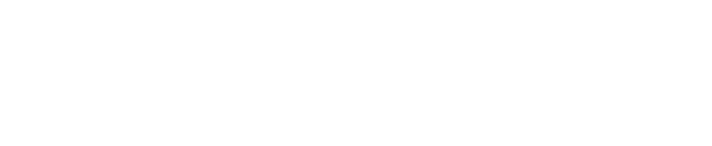
We are a management consultancy applying behavioral insights to create business and societal impact. We use our expertise in behavioral economics and social psychology to design evidence-based solutions to critical challenges. We leverage scientific methods to identify interventions that will have long lasting, measurable effects.
DISCLAIMER
FOLLOW US
CONTACT
Email: info@impactually.se
Org. no.: 559167-0327
Web Design: Sharp Studio
Copyright Impactually 2024